Monetizing GenAI: Navigating the Future of AI Infrastructure
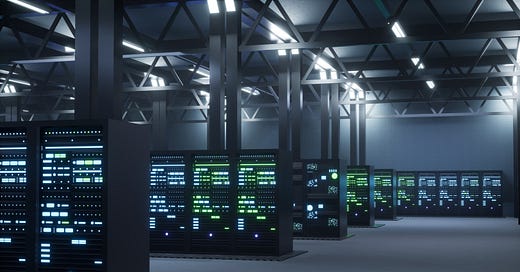
The rapid evolution of Generative AI has unlocked new possibilities for industries, ranging from personalized customer support to intelligent automation and advanced data analytics. However, despite the growing demand for these cutting-edge technologies, effectively monetizing them, especially when it comes to their underlying infrastructure, remains a significant challenge. The recent developments with OpenAI, particularly around their ChatGPT Pro subscriptions, provide valuable lessons for other AI service providers on the complexities of monetization in the GenAI space.
Sam Altman recently disclosed that OpenAI is losing money on ChatGPT Pro subscriptions, despite their widespread popularity, due to higher-than-expected usage. This is a critical issue that highlights the financial strain AI providers face, primarily due to the operational costs of running large-scale models like GPT-4, which require immense computational power and substantial financial investment. For investors and founders alike, OpenAI’s predicament serves as a cautionary tale, signaling the need for a more sustainable and adaptable monetization strategy.
The Hybrid Monetization Model: A Strategic Approach for AI Infrastructure
To mitigate the financial challenges and increase profitability, the future of GenAI monetization lies in adopting a hybrid monetization model that effectively combines the stability of traditional data centers with the scalability of hyperscalers (cloud providers). This model not only optimizes resource utilization but also accommodates the fluctuating demand of GenAI services.
Key Components of the Hybrid Model:
- Dynamic Capacity Planning:
- Traditional Data Centers: These are ideal for managing predictable, steady-state workloads. They allow providers to optimize their investments in physical infrastructure by ensuring that resources are available without overextension. However, traditional data centers struggle with spikes in demand.
- Hyperscalers: Cloud infrastructure providers like AWS, Google Cloud, and Azure are designed to handle elastic, scalable workloads. They provide on-demand computing resources to accommodate sudden spikes in GenAI demand, leveraging their vast data centers spread across regions. This scalability is essential for handling the growing needs of AI models, which require more processing power as they become more sophisticated.
- Integrated Billing Systems:
- Unified Billing Platforms: Solutions such as Monetize360 enable AI service providers to implement complex billing structures that align with the evolving usage patterns of GenAI services. These systems can handle subscription-based models, usage-based pricing, or a hybrid approach, allowing flexibility in pricing while ensuring transparency in customer transactions. With the rise of AI-as-a-Service (AIaaS) offerings, unified billing systems are crucial for managing diverse pricing schemes across different customer segments and geographies.
The Rise of "AI-as-a-Service" (AIaaS) and its Impact on Monetization
A new trend in the GenAI landscape is the rise of AI-as-a-Service (AIaaS), where companies offer advanced AI capabilities on-demand through cloud platforms. The proliferation of AI tools has made this model attractive to a broader range of industries, reducing the barrier to entry for businesses looking to integrate AI without heavy upfront costs. For AI providers, monetizing AIaaS effectively requires a nuanced pricing approach, combining subscription-based access with pay-as-you-go models for high-demand services.
- Consumption-Based Models: The increasing complexity of AI models and the computational cost of running them create an opportunity for usage-based pricing models. Rather than charging a flat fee, providers can charge customers based on how much processing power they consume, how many queries are run, or the volume of data processed. This model enables providers to scale revenue in line with usage, helping to avoid the losses OpenAI experienced with ChatGPT Pro.
- Tiered Pricing: Offering tiered pricing based on performance (e.g., response time or feature set) and usage volume allows customers to pay according to their needs. AI models can be offered with varying levels of speed, capacity, or sophistication, allowing companies to better monetize their offerings based on demand elasticity.
Lessons from OpenAI’s Experience: Real-World Monetization Challenges
OpenAI’s losses on their ChatGPT Pro subscriptions underscore the importance of aligning pricing strategies with the high costs of running AI models. As AI models such as GPT-4 become more advanced, their demand for computational resources grows exponentially. In fact, the cost of inference—the process of making predictions or generating outputs from trained AI models—can be prohibitive, particularly when scaled to meet the needs of a global user base.
The key takeaway here is that overestimating potential revenue without factoring in the high computational expenses associated with running advanced GenAI models can create significant financial strain. AI service providers must build more robust capacity planning and pricing models that account for operational costs and fluctuating demand.
Strategies for Sustainable Monetization in GenAI
- Usage-Based Pricing: Aligning fees with actual consumption helps ensure that revenue scales with usage. This mitigates the risk of losses due to sudden spikes in demand or unforeseen customer consumption patterns.
- Scalable Infrastructure Investments: Given that GenAI models require substantial processing power, investing in scalable infrastructure is crucial. Providers must leverage cloud-based infrastructure, especially hyperscalers, to handle unexpected surges in demand, while maintaining control over operational costs.
- Advanced Billing Solutions: Adopting billing platforms that handle complex AI services, such as dynamic pricing based on usage or AI models' computational intensity, can provide clarity and flexibility for both customers and providers. These platforms can enable real-time billing adjustments to ensure that AI usage is billed based on actual consumption, enhancing revenue predictability and transparency.
Conclusion: A Roadmap for Sustainable AI Monetization
The challenges faced by OpenAI serve as a wake-up call for AI service providers, particularly those building on large-scale infrastructure. As investors, it's critical to recognize that the monetization of GenAI services requires a careful balance between technological capabilities and sustainable pricing models. By adopting a hybrid approach to infrastructure, embracing usage-based pricing, and leveraging advanced billing solutions, AI service providers can better manage costs and unlock scalable revenue opportunities.
For data centers and cloud providers navigating the complexities of GenAI, strategic investments in both infrastructure and billing models will be key to ensuring profitability and long-term growth. With a strategic approach, GenAI can not only transform industries but also drive sustainable and profitable growth for service providers in the years to come.